BloombergGPT is going to replace the analyst
Analysts are fundamentally chat-based interfaces that senior finance professionals use to gather, organize, and output data
Forget about sentiment analysis, stock price prediction, and anything else that might be perceived as “alpha” generative. Large language models (LLMs) will disrupt financial services, but not because they’ll generate alpha. BloombergGPT won’t replace the partner. BloombergGPT will replace the analyst.
Turning comments is the bane of high finance
Unless you’ve worked in high finance - specifically, banking, consulting, and private equity - you likely have a distorted perception of how a financial analyst spends his time. Movies like Wall Street, The Big Short, and The Wolf of Wall Street portray days spent debating investment theses, conducting research in the field, and plotting against adversarial counterparties. In reality, a financial analyst spends about 5% of his time “wheeling and dealing,” and 95% of his time “turning comments.”
For the uninitiated, “turning comments” is finance jargon for the process of iterating on an output related to a deal process. Outputs are typically PowerPoint slides with content that ranges from simple bullet points detailing an investment’s risks, to screenshots of a complex Excel model. The comments range similarly from a quick text change, to a complete overhaul of the Excel model. Almost every output produced in finance is done in an iterative manner up and down the deal team.
To start, the partner requests an output such as a chart for a PowerPoint slide. The analyst attempts to create this chart and sends his first draft to the VP. The VP requests changes based on what she thinks the partner wants to see. The analyst updates his draft based on these changes (i.e., “turns the comments”) and sends it back to the VP. The VP and analyst continue this iterative loop until the VP decides the chart is good enough to send to the partner. At this stage, the partner either accepts the chart or requests his own edits which restarts the aforementioned loop. One might say the partner prompts the deal team and then iterates with follow-up prompts until he is satisfied with the response.
When considering the aggregate work done by front-office professionals in a typical financial firm, the process of turning comments consumes most of the man hours.
Primary stages of a deal process
There are 3 main stages in any deal process:
Generate Idea: A partner has an idea for something revenue generative. Likely struck by divine intervention, he hypothesizes his portfolio company Viagogo can make a lot of money by acquiring its rival StubHub.
Create Outputs: His idea is just a hypothesis. He needs data to back it up. He’ll want at least 60 slides covering everything from competitive benchmarking to exit multiple analysis. He sends his request to the VP and analyst who immediately begin creating outputs and turning comments.
Make Decision: The outputs look good and his hypothesis was validated. He makes a decision. Viagogo will acquire its rival StubHub. He conveys his message to the rest of the firm and excitedly awaits his inevitable score.
Constructing the model firm
Our model firm is going to be comprised of 5 partners that are cumulatively working on 40 deals at any given time. For each deal, the deal partner builds a deal team. A deal team is comprised of 3 members: a partner, a vice president, and an analyst.
The Partner is the most senior member of the deal team. His main job is to generate new ideas (i.e., M&A opportunities, strategic initiatives, take-privates), and to make decisions about those ideas (i.e., “Will this make the firm lots of money?”). The partner is the source of all output requests. He needs these outputs to help him make decisions, and to convince other stakeholders of those decisions. He might hypothesize that his deal will generate lots of profits, but he needs the VP and analyst to collect, synthesize, and output the data so that he can make his decision.
The Vice President is the middle manager. Her main job is to manage the creation of outputs. She serves as the partner’s regent and directs the analyst’s output creation. She’s likely worked with the partner for a number of years and has a sense for his personal preferences — What metrics does he like to see on a sensitivity table? How does he like to see ARR bridges formatted? What precedent transactions would he want included on the exit multiple page? While she might not perfectly reflect the partner’s specific preferences, it’s easier for him to let the VP guide the outputs from the 20% v1 solution to the 80% v10 solution.
The Analyst is the most junior member of the deal team. His main job is to create the outputs. He collects data from disparate sources such as company filings, industry reports, and even phone calls with customers. He then cleans this data, synthesizes it into the important takeaways, and then presents it in the form requested by the partner.
If you drop our 3 archetypal team members into the 3 stages of a deal process, you get something like this:
Simplifying the processes a bit, we can distill all time spent by members of this model firm into two basic categories:
Doing Deals: This encompasses the idea generation and decision making that is the primary responsibility of the partner. That being said, all members of the deal team spend some percentage of their time on these higher-order activities.
Creating Outputs: This encompasses the collecting, synthesizing, and outputting data required to guide decision making and convince relevant stakeholders. This is primarily the role of the analyst, but all members of the deal team spend some percentage of their time on these lower-order activities.
To finish up our model, let’s make a few more assumptions. There are 5 partners who work 40-hour workweeks, spend 90% of their time doing deals, and process 8 concurrent deals each at any given time. There are 10 VPs who work 60-hour workweeks, spend 70% of their time creating outputs, and process 4 concurrent deals each. There are 20 analysts who work 80-hour workweeks, spend 95% of their time creating outputs, and process 2 concurrent deals each.
We can wrap everything up in the following table:
There is one very important takeaway: Over 80% of total man hours in this model firm are spent creating outputs.
Why does this even matter?
I can assure you that time spent turning comments and creating outputs is not fun. Even worse, it doesn’t generate any alpha. Investment outperformance is driven by making better decisions than the next guy. All time spent creating outputs takes away from time spent analyzing those outputs and thinking about decisions.
To be specific, there is a correct way to build an LBO model. In fact, there are many correct ways to build an LBO, however, nobody makes money by building a correct LBO model. People make money by using a correct LBO model to make better decisions about investment opportunities.
How can financial professionals spend less time building LBO models and more time making investment decisions?
I’ll give you one guess…
What’s BloombergGPT?
BloombergGPT is a large language model (LLM) trained on a dataset comprised of 50% financial data and 50% the entire internet. Large language models use fancy math to approximate natural language allowing you to chat with them like they were humans.
Basically, BloombergGPT is ChatGPT but trained to be better at responding to finance-specific prompts. If your knowledge of ChatGPT is fuzzy, I urge you to sign up for free and spend 5 minutes playing with it. This technological innovation warrants 5 minutes of hands-on learning from even the most devout luddites and techno skeptics. To go in a bit of depth on how LLMs work, watch this YouTube series.
BloombergGPT is a chat-based interface used to gather, organize, and output financial data.
How will BloombergGPT replace the analyst?
An analyst is a chat-based interface used to gather, organize, and output financial data.
An analyst accomplishes the same role as a finance-focused large language model. 95% of an analyst’s time is spent creating outputs. Doing so is almost entirely comprised of gathering, organizing, and outputting data.
His main job is to create the outputs. He collects data from disparate sources such as company filings, industry reports, and even phone calls with customers. He then cleans this data, synthesizes it into the important takeaways, and then presents it in the form requested by the partner.
Additionally, analysts are already chat-based interfaces. Put simply, we get analysts to do their jobs by chatting with them. If a partner wants to see a slide with a waterfall chart, he emails the analyst: “Please present the target company’s 2022 Annual Recurring Revenue in a waterfall chart.” If the first draft of the output isn’t quite what the partner wants, he responds by sending additional comments via email: “Please change the colors to match our firm’s official color palette.”
Imagine the analyst as a software application designed to create financial outputs. The partner doesn’t even need to change his workflow. Instead of emailing van@spina-securities.com, the partner just emails vanGPT@spina-securities.com. Even if the output is worse than what the human Van could prepare, the partner will prefer VanGPT. Using a large language model massively cuts down iteration timelines.
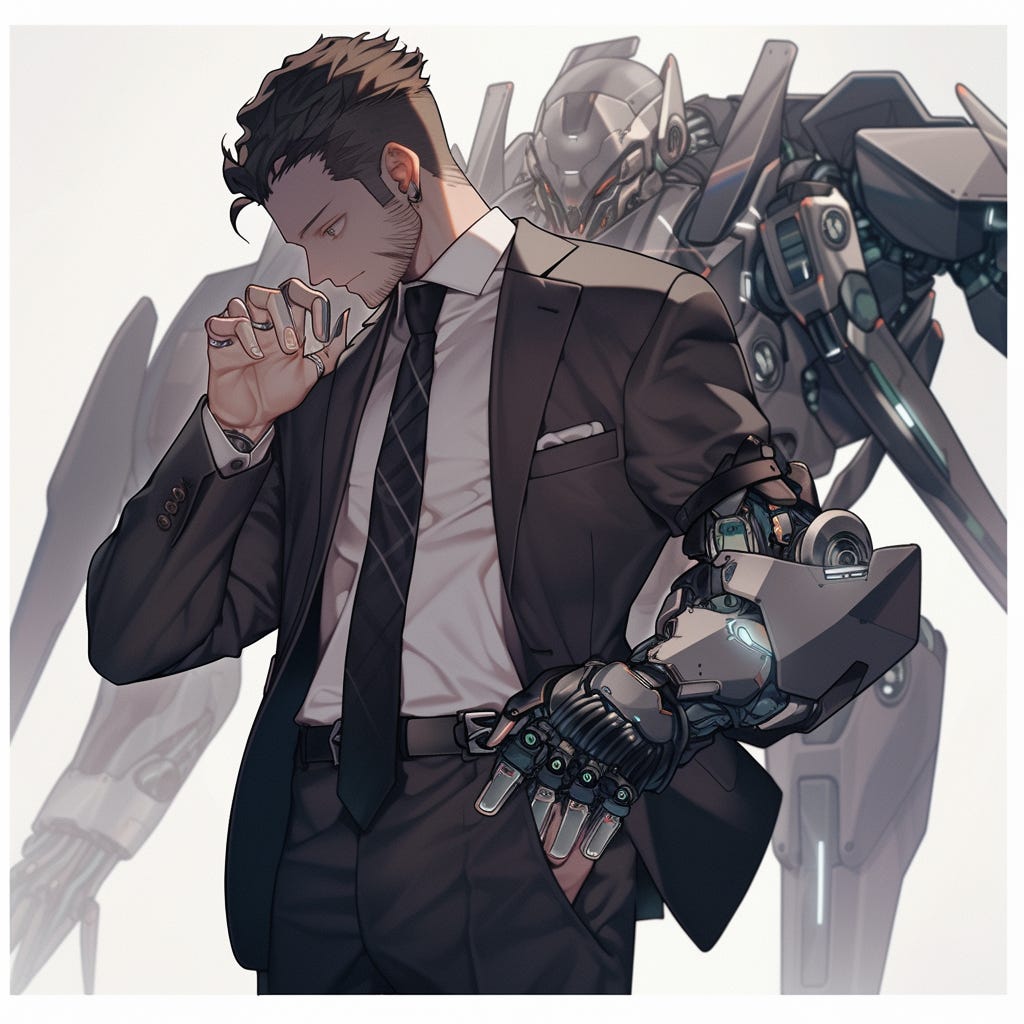
The biggest advantage of using a large language model is that it responds in seconds. If an analyst is creating a new output using Excel and PowerPoint, he has to manually click and type. Even if he’s a rockstar analyst with no mouse and 5 keys removed for efficiency, the creation of a de novo LBO model for a new deal will take at least an hour. Let’s say the partner wants to add a dividend recap after the v1 model was delivered. Now you’re tacking on another 30 minutes. If two partners have modeling requests at the same time from the same analyst, there is no option but to complete them sequentially. The partner isn’t getting v2 of his model for at least 3 hours. Analyst time does not scale well because creating outputs is highly manual. This is what drives the pyramid-shaped headcount chart in our model firm table.
Using BloombergGPT, the same partner can iterate 50 times in 5 minutes. Even compared to the most efficient deal team ever, he’s getting back to making decisions 1.5 to 3 hours faster. In reality, the delays in creating outputs are meaningfully longer.
A more likely scenario would be as follows:
Monday, 2pm: Partner decides he wants to see take-private math on Twitter. He emails the VP his request.
Monday, 2:30pm: VP checks her email. She immediately calls the analyst and requests a version of the model as soon as possible.
Tuesday, 9:30am: Analyst begins building the model. He didn’t start on Monday because he had to finish quarterly reporting due that night.
Tuesday, 4pm: Analyst sends model to VP.
Tuesday, 6pm: VP gets out of a board meeting and skims the model. She realizes the analyst has an error in the debt paydown calc. She sends the model back.
…more turning comments, more iteration, etc. etc. etc.
Friday, 5pm: Partner finally receives a slide deck outlining the take-private math on Twitter.
This is when the next tricky situation arrives. The partner knows the analyst and VP have been toiling over this model and working long nights all week to create the complicated set of outputs. But now it’s 5pm on a Friday, bids are due Monday, and the partner has another question. How does the math change if Twitter rolls out Twitter Blue at $8/month? This isn’t currently factored into the model so he needs to see another iteration. He needs to send more comments, but he hesitates…
He knows the analyst has a “protected Saturday” and is already in flight to visit college friends in Washington D.C. Furthermore, the VP is at her old roommate’s wedding on the West Coast.
Iterating on a model and re-outputting the deck is not an idle activity. The analyst and VP can’t do it from their phones. They need to be on their laptops, and the analyst probably wants to plug into dual monitors. Deals don’t sleep and this analysis is critical. The partner begrudgingly asks to see the revised analysis by Sunday morning.
Sunday morning rolls around, he opens his email and checks the outputs. The deal doesn’t pencil. It was all for naught.
In a world where output creation is done by large language models, the partner received a terrible v1 on Monday at 2:01pm. By Monday at 2:17pm, he has a complete set of outputs and already realizes the deal doesn’t pencil. At 2:20pm, he re-runs the math with Twitter Blue. Still no luck.
At 2:43pm, he wonders how the math will look if he buys Twitter and Dogecoin in equal size and convinces Elon to change Twitter’s logo to a Doge. He re-prompts the LLM. It’s a complicated analysis with many levers, but by 3:11pm, he’s iterated to a point where he’s happy with the outputs. He jumps to the final page. It’s accretive.
An example: creating outputs with ChatGPT
Large language models aren’t yet quite sophisticated enough to completely replace the analyst. Even BloombergGPT is really just a proof of concept that demonstrates training models to be domain-specific can result in better performance. It does do some cool things like enable users to generate Bloomberg Query Language search prompts using natural language, but it’s not building a full set of outputs for a Twitter LBO in 19 minutes. That being said, this technology is moving quickly.
A common output created when assessing software companies is the waterfall chart. It shows beginning ARR, net new sales, upsell, downsell, churn, and ending ARR but in an intuitive way that makes it easy to see the summation. Without using a Microsoft Office plug-in like ThinkCell, it’s an absolute nightmare to create by hand. A YouTube tutorial titled “Easiest Excel Waterfall Chart” is literally 33 minutes long.
As an experiment, I asked ChatGPT to create a waterfall chart for me. Out of the box, ChatGPT doesn’t port into Excel or PowerPoint, but it is pretty good at writing code. As a workaround, I asked ChatGPT to write a simple script that displays a waterfall chart when run. I added a few specifications about the axes and values, and submitted my prompt.
It decided to write the script in Python and even imported a third-party library called Plotly to help with the chart. I dropped the code in Replit, a web-based development environment, and hit run.
No luck. I ran into an error but could tell I was close. Like any good financial professional, I provided some comments to my new AI-based analyst.
After a few rounds of turning comments, I received this awesome AI-generated waterfall chart that leveraged Python, Plotly, Flask, and Replit to create a web app.
I then provided one more comment to apply the company’s official color palette — Cyberpunk. If you’d like to check out the final product or read the chat logs, here are some links:
Chat Logs if you want to see what it’s like to iterate on an output with ChatGPT (Please check out the logs if you’ve never used ChatGPT before)
Web App for the waterfall chart (It will be slow because it’s not deployed)
Replit Repl if you want to check out the source code
What does this mean for our model firm?
There were 100 summer analysts in my investment banking intern class. We all spent our summer creating outputs. Finance will need fewer analysts, and fewer VPs to manage them.
Let’s assume large language models handle 100% of the output creation and deal teams spend 100% of their time doing deals. If we turn back to our model firm, you’ll see we’ve flipped the pyramid on it’s head.
Our firm has gone from 35 people to 9 people but is still managing the same amount of dealflow.
Our 5 partners are still working on their 8 concurrent deals each, but now they’re leaving at 4pm instead of 5pm and they’ve started taking tennis lessons.
Our vice presidents are still working 60-hour weeks but spending their time entirely on higher-order activities. They’re handling 13 concurrent deals and their ranks have been cut from 10 to 3 VPs.
Finally, there’s 1 analyst remaining. He’s getting some great reps under his belt and seeing a lot of action working all 40 of the firm’s deals. If he makes it all the way to partner, he’ll have a hell of a lot of valuable experience to draw from.
Are current and prospective analysts doomed?
Historically, if you wanted to get promoted in finance, you needed to start doing your boss’s job. If you’re an analyst today, doing your boss’s job might not get you promoted anymore. You need to start doing your boss’s boss’s job. You need to start generating ideas and making decisions.
Well, maybe not making decisions yet. But you need to show you’re the type who will one day create dealflow and make profitable decisions. Keyboard speed and ability to “burn the midnight oil” will become less and less differentiating as LLMs creep into modern financial workflows.
Additionally, I don’t think the pyramid will actually flip. If there are 5 partners, maybe there will be 7 VPs and 10 analysts. These firms don’t just hire to meet workload capacity, they also hire for succession planning. Besides, someone needs to man the ship at headquarters when the partners are out-of-office. Those mountains in aspen aren’t gonna ski themselves, that party in saint-tropez isn’t gonna enjoy itself, that kiteboard on necker island needs someone to fly it, etc etc etc.
A few ending notes
As Mark Twain said, “I didn’t have time to write a short letter, so I wrote a long one instead.” If you made it this far, thanks for reading and please consider subscribing.
Shoutout to Anand Sanwal, Joshua Benton,
, and Angel Au-Yeung for engaging with my original tweet on this topic. The number of impressive people my tweet reached inspired me to both write this post, and to start a Substack in general. It's cool when your ideas reach people you otherwise wouldn't be able to engage with.If you’re a member of the BloombergGPT team or OpenAI team and want to brainstorm about ways to tailor these products for financial workflows, my Twitter DMs are open. I have plenty more ideas.
Finally, let me know in the comments below how long you think it will be until AI takes over output creation in finance, and how long until it moves further up the chain to start generating alpha directly.
This is fantastic, super informative, thanks Van!
Love it Van. Hope all is well!